References
Akçakaya, M., Yaman, B., Chung, H., Ye, J. C., 2021. Unsupervised deep learning methods for biological image reconstruction arXiv:2105.08040.
Allan, T. W., Francis, S. T., Caballero-Gaudes, C., Morris, P. G., Liddle, E. B., Liddle, P. F., … Gowland, P. A. (2015). Functional Connectivity in MRI Is Driven by Spontaneous BOLD Events. PLOS ONE, 10(4), e0124577. https://doi.org/10.1371/JOURNAL.PONE.0124577
Andersson, J. L., Skare, S., Ashburner, J., 2003. How to correct susceptibility distortions in spinecho echo-planar images: application to diffusion tensor imaging. NeuroImage 20, 870–888. doi:10.1016/s1053–8119(03)00336–7.
Aslan, S., Cemgil, A. T., Akin, A., 2016. Joint state and parameter estimation of the hemodynamic model by particle smoother expectation maximization method. Journal of Neural Engineering 13, 046010. doi:10.1088/1741–2560/13/4/046010.
Beck, A., & Teboulle, M. (2009). A Fast Iterative Shrinkage-Thresholding Algorithm for Linear Inverse Problems. SIAM Journal on Imaging Sciences, 2(1), 183–202. https://doi.org/10.1137/080716542
Behzadi, Y., Restom, K., Liau, J., & Liu, T. T. (2007). A component based noise correction method (CompCor) for BOLD and perfusion based fMRI. NeuroImage, 37(1), 90–101. https://doi.org/10.1016/J.NEUROIMAGE.2007.04.042
Birn, R. M., Diamond, J. B., Smith, M. A., Bandettini, P. A., 2006. Separating respiratory-variation-related fluctuations from neuronal-activity-related fluctuations in fMRI. NeuroImage 31, 1536–1548. doi:10.1016/j.neuroimage.2006.02.048.
Bolton, T. A., Morgenroth, E., Preti, M. G., Ville, D. V. D., 2020. Tapping into multi-faceted human behavior and psychopathology using fMRI brain dynamics. Trends in Neurosciences 43, 667–680. doi:10.1016/j.tins.2020.06.005.
Bommarito, G., Tarun, A., Farouj, Y., Preti, M. G., Petracca, M., Droby, A., Mendili, M. M. E., Inglese, M., Ville, D. V. D., 2020. Altered anterior default mode network dynamics in progressive multiple sclerosis. medRxiv doi:10.1101/2020.11.26.20238923.
Bommarito, G., Tarun, A., Farouj, Y., Preti, M. G., Petracca, M., Droby, A., Mounir El Mendili, M., Inglese, M., Van De Ville, D., in press. Altered anterior default mode network dynamics in progressive multiple sclerosis. Multiple Sclerosis Journal doi:10.1177/13524585211018116.
Boynton, G. M., Engel, S. A., Glover, G. H., Heeger, D. J., 1996. Linear systems analysis of functional magnetic resonance imaging in human v1. The Journal of Neuroscience 16, 4207–4221. doi:10.1523/jneurosci.16–13–04207.1996.
Bruckstein, A.M., Donoho, D. L., Elad, M., 2009. From sparse solutions of systems of equations to sparse modeling of signals and images. SIAM Review 51, 34–81. doi:10.1137/060657704.
Bush, K., Cisler, J., 2013. Decoding neural events from fMRI BOLD signal: A comparison of existing approaches and development of a new algorithm. Magnetic Resonance Imaging 31, 976–989. doi:10.1016/j.mri.2013.03.015.
Bush, K., Zhou, S., Cisler, J., Bian, J., Hazaroglu, O., Gillispie, K., Yoshigoe, K., Kilts, C., 2015. A deconvolution-based approach to identifying large-scale effective connectivity. Magnetic Resonance Imaging 33, 1290–1298. doi:10.1016/j.mri.2015.07.015.
Buxton, R. B., Wong, E. C., Frank, L. R., 1998. Dynamics of blood flow and oxygenation changes during brain activation: the balloon model. Magnetic resonance in medicine 39, 855–864.
Caballero-Gaudes, C., Moia, S., Panwar, P., Bandettini, P. A., Gonzalez-Castillo, J., 2019. A deconvolution algorithm for multi-echo functional MRI: Multi-echo sparse paradigm free mapping. NeuroImage 202, 116081. doi:10.1016/j.neuroimage.2019.116081.
Casanova, R., Ryali, S., Serences, J., Yang, L., Kraft, R., Laurienti, P. J., Maldjian, J. A., 2008. The impact of temporal regularization on estimates of the BOLD hemodynamic response function: A comparative analysis. NeuroImage 40, 1606–1618. doi:10.1016/j.neuroimage.2008.01.011.
Chambolle, A., 2004. An algorithm for total variation minimization and applications. Journal of Mathematical imaging and vision 20, 89–97.
Chen, J. E., Glover, G. H., Fultz, N. E., Rosen, B. R., Polimeni, J. R., Lewis, L. D., 2021. Investigating mechanisms of fast BOLD responses: The effects of stimulus intensity and of spatial heterogeneity of hemodynamics. NeuroImage 245, 118658. doi:10.1016/j.neuroimage.2021.118658.
Chen, S. S., Donoho, D. L., Saunders, M. A., 2001. Atomic decomposition by basis pursuit. SIAM review 43, 129–159.
Cherkaoui, H., Moreau, T., Halimi, A., Ciuciu, P., 2019. Sparsity-based blind deconvolution of neural activation signal in FMRI, in: ICASSP 2019 - 2019 IEEE International Conference on Acoustics, Speech and Signal Processing (ICASSP), IEEE. pp. 1323–1327. doi:10.1109/icassp.2019.8683358.
Cherkaoui, H., Moreau, T., Halimi, A., Leroy, C., Ciuciu, P., 2020a. Multivariate semi-blind deconvolution of fMRI time series. URL: https://hal.archives-ouvertes.fr/hal–03005584. working paper or preprint.
Cherkaoui, H., Sulam, J., Moreau, T., 2020b. Learning to solve TV regularised problems with unrolled algorithms 33, 11513–11524. URL: https://proceedings.neurips.cc/paper/2020/file/84fec9a8e45846340fdf5c7c9f7ed66c-Paper.pdf.
Cifre, I., Flores, M. T. M., Ochab, J. K., Chialvo, D. R., 2020a. Revisiting non-linear functional brain co-activations: directed, dynamic and delayed. arXiv:2007.15728.
Cifre, I., Zarepour, M., Horovitz, S. G., Cannas, S. A., Chialvo, D. R., 2020b. Further results on why a point process is effective for estimating correlation between brain regions. Papers in Physics 12, 120003. doi:10.4279/pip.120003.
Ciuciu, P., Poline, J. B., Marrelec, G., Idier, J., Pallier, C., Benali, H., 2003. Unsupervised robust nonparametric estimation of the hemodynamic response function for any fmri experiment. IEEE transactions on medical imaging 22, 1235–1251. doi:10.1109/TMI.2003.817759.
Cohen, M. S., 1997. Parametric analysis of fMRI data using linear systems methods. NeuroImage 6, 93–103. doi:10.1006/nimg.1997.0278.
Costantini, I., Deriche, R., Deslauriers-Gauthier, S., 2022. An anisotropic 4d filtering approach to recover brain activation from paradigm-free functional MRI data. Frontiers in Neuroimaging 1. doi: 10.3389/fnimg.2022.815423.
Cox, R. W., 1996. AFNI: Software for analysis and visualization of functional magnetic resonance neuroimages. Computers and Biomedical Research 29, 162–173. doi:10.1006/cbmr.1996.0014.
Deco, G., Kringelbach, M. L., 2017. Hierarchy of information processing in the brain: A novel ‘intrinsic ignition’ framework. Neuron 94, 961–968. doi:10.1016/j.neuron.2017.03.028.
Deco, G., Tagliazucchi, E., Laufs, H., Sanjuán, A., Kringelbach, M. L., 2017. Novel intrinsic ignition method measuring local-global integration characterizes wakefulness and deep sleep. eNeuro 4. doi:10.1523/ENEURO.0106–17.2017.
Di, X., Biswal, B. B., 2013. Modulatory interactions of resting-state brain functional connectivity. PLoS ONE 8, e71163. doi:10.1371/journal.pone.0071163.
Di, X., Biswal, B. B., 2015. Characterizations of resting-state modulatory interactions in the human brain. Journal of Neurophysiology 114, 2785–2796. doi:10.1152/jn.00893.2014.
Di, X., Biswal, B. B., 2018. Toward task connectomics: Examining whole-brain task modulated connectivity in different task domains. Cerebral Cortex 29, 1572–1583. doi:10.1093/cercor/bhy055.
Efron, B., Hastie, T., Johnstone, I., Tibshirani, R., 2004. Least angle regression. The Annals of Statistics 32, 407–499. doi:10.1214/009053604000000067.
Elad, M., Milanfar, P., Rubinstein, R., 2007. Analysis versus synthesis in signal priors. Inverse Problems 23, 947–968. doi:10.1088/0266–5611/23/3/007.
Esfahlani, F. Z., Jo, Y., Faskowitz, J., Byrge, L., Kennedy, D. P., Sporns, O., Betzel, R. F., 2020. High-amplitude cofluctuations in cortical activity drive functional connectivity. Proceedings of the National Academy of Sciences 117, 28393–28401. doi:10.1073/pnas.2005531117.
Farouj, Y., Karahanoglu, F. I., Ville, D. V. D., 2019. Bold signal deconvolution under uncertain hÆmodynamics: A semi-blind approach, in: 2019 IEEE 16th International Symposium on Biomedical Imaging (ISBI 2019), IEEE. IEEE. pp. 1792–1796. doi:10.1109/isbi.2019.8759248.
Farouj, Y., Karahanoglu, F. I., Ville, D. V. D., 2020. Deconvolution of sustained neural activity from large-scale calcium imaging data 39, 1094–1103. doi:10.1109/tmi.2019.2942765.¶
Faskowitz, J., Esfahlani, F. Z., Jo, Y., Sporns, O., Betzel, R. F., 2020. Edge-centric functional network representations of human cerebral cortex reveal overlapping system-level architecture. Nature neuroscience 23, 1644–1654. doi:10.1038/s41593–020–00719-y.
Feinberg, D. A., Moeller, S., Smith, S. M., Auerbach, E., Ramanna, S., Glasser, M. F., Miller, K. L., Ugurbil, K., Yacoub, E., 2010. Multiplexed echo planar imaging for sub-second whole brain FMRI and fast diffusion imaging. PLoS ONE 5, e15710. doi:10.1371/journal.pone.0015710.
Freitas, L. G., Bolton, T. A., Krikler, B. E., Jochaut, D., Giraud, A. L., Hüppi, P. S., Ville, D. V. D., 2020. Time-resolved effective connectivity in task fMRI: Psychophysiological interactions of co-activation patterns. NeuroImage 212, 116635. doi:10.1016/j.neuroimage.2020.116635.
Friedrich, J., Zhou, P., Paninski, L., 2017. Fast online deconvolution of calcium imaging data. PLOS Computational Biology 13, e1005423. doi:10.1371/journal.pcbi.1005423.
Friston, K., Fletcher, P., Josephs, O., Holmes, A., Rugg, M., Turner, R., 1998. Event-related fMRI: Characterizing differential responses. NeuroImage 7, 30–40. doi:10.1006/nimg.1997.0306.
Friston, K., Trujillo-Barreto, N., Daunizeau, J., 2008. DEM: A variational treatment of dynamic systems. NeuroImage 41, 849–885. doi:10.1016/j.neuroimage.2008.02.054.
Friston, K. J., Jezzard, P., Turner, R., 1994. Analysis of functional MRI time-series. Human Brain Mapping 1, 153–171. doi:10.1002/hbm.460010207.
Friston, K. J., Mechelli, A., Turner, R., Price, C. J., 2000. Nonlinear responses in fmri: the balloon model, volterra kernels, and other hemodynamics. NeuroImage 12, 466–477.
Gaudes, C. C., Karahanoglu, F. I., Lazeyras, F., Ville, D. V. D., 2012. Structured sparse deconvolution for paradigm free mapping of functional MRI data, in: 2012 9th IEEE International Symposium on Biomedical Imaging (ISBI), IEEE. IEEE. pp. 322–325. doi:10.1109/isbi.2012.6235549.
Gaudes, C. C., Petridou, N., Dryden, I. L., Bai, L., Francis, S. T., Gowland, P. A., 2010. Detection and characterization of single-trial fMRI bold responses: Paradigm free mapping. Human Brain Mapping 32, 1400–1418. doi:10.1002/hbm.21116.
Gaudes, C. C., Petridou, N., Francis, S. T., Dryden, I. L., Gowland, P. A., 2013. Paradigm free mapping with sparse regression automatically detects single-trial functional magnetic resonance imaging blood oxygenation level dependent responses. Human Brain Mapping, n/a–n/adoi:10.1002/hbm.21452.
Gaudes, C. C., Van De Ville, D., Petridou, N., Lazeyras, F., Gowland, P., 2011. Paradigm-free mapping with morphological component analysis: Getting most out of fmri data, in: Wavelets and Sparsity XIV, International Society for Optics and Photonics. p. 81381K.
Gerchen, M. F., Bernal-Casas, D., Kirsch, P., 2014. Analyzing task-dependent brain network changes by whole-brain psychophysiological interactions: A comparison to conventional analysis. Human Brain Mapping 35, 5071–5082. doi:10.1002/hbm.22532.
Gitelman, D. R., Penny, W. D., Ashburner, J., Friston, K. J., 2003. Modeling regional and psychophysiologic interactions in fMRI: the importance of hemodynamic deconvolution. NeuroImage 19, 200–207. doi:10.1016/s1053–8119(03)00058–2.
Glasser, M. F., Smith, S. M., Marcus, D. S., Andersson, J. L. R., Auerbach, E. J., Behrens, T. E. J., Coalson, T. S., Harms, M. P., Jenkinson, M., Moeller, S., Robinson, E. C., Sotiropoulos, S. N., Xu, J., Yacoub, E., Ugurbil, K., Essen, D.C. V., 2016. The human connectome project’s neuroimaging approach. Nature Neuroscience 19, 1175–1187. doi:10.1038/nn.4361.
Glover, G. H., 1999. Deconvolution of impulse response in event-related BOLD fMRI1. NeuroImage 9, 416–429. doi:10.1006/nimg.1998.0419.
Gonzalez-Castillo, J., Caballero-Gaudes, C., Topolski, N., Handwerker, D. A., Pereira, F., Bandettini, P. A., 2019. Imaging the spontaneous flow of thought: Distinct periods of cognition contribute to dynamic functional connectivity during rest. NeuroImage 202, 116129. doi:10.1016/j.neuroimage.2019.116129.
Gonzalez-Castillo, J., Saad, Z. S., Handwerker, D. A., Inati, S. J., Brenowitz, N., Bandettini, P. A., 2012. Whole-brain, time-locked activation with simple tasks revealed using massive averaging and model-free analysis. Proceedings of the National Academy of Sciences of the United States of America 109, 5487–5492. doi:10.1073/pnas.1121049109.
Goutte, C., Nielsen, F. A., Hansen, L. K., 2000. Modeling the haemodynamic response in fmri using smooth fir filters. IEEE transactions on medical imaging 19, 1188–1201. doi:10.1109/42.897811.
Havlicek, M., Friston, K. J., Jan, J., Brazdil, M., Calhoun, V. D., 2011. Dynamic modeling of neuronal responses in fMRI using cubature kalman filtering. NeuroImage 56, 2109–2128. doi:10.1016/j.neuroimage.2011.03.005.
Henson, R., Friston, K., 2007. Chapter 14 - convolution models for fmri, in: Friston, K., Ashburner, J., Kiebel, S., Nichols, T., Penny, W. (Eds.), Statistical Parametric Mapping. Academic Press, London, pp. 178–192. URL: https://www.sciencedirect.com/science/article/pii/B9780123725608500140, doi:https://doi.org/10.1016/B978–012372560–8/50014–0.
Hernandez-Garcia, L., Ulfarsson, M. O., 2011. Neuronal event detection in fMRI time series using iterative deconvolution techniques. Magnetic Resonance Imaging 29, 353–364. doi:10.1016/j.mri.2010.10.012.
Hütel, M., Antonelli, M., Melbourne, A., Ourselin, S., 2021. Hemodynamic matrix factorization for functional magnetic resonance imaging. NeuroImage 231, 117814. doi:10.1016/j.neuroimage.2021.117814.
Hüitel, M., Melbourne, A., Ourselin, S., 2018. Neural activation estimation in brain networks during task and rest using BOLD-fMRI, in: Medical Image Computing and Computer Assisted Intervention—MICCAI 2018, Springer. Springer International Publishing. pp. 215–222. doi:10.1007/978–3–030–00931–1_25.
Jenkinson, M., Beckmann, C. F., Behrens, T. E., Woolrich, M. W., Smith, S. M., 2012. FSL. NeuroImage 62, 782–790. doi:10.1016/j.neuroimage.2011.09.015.
Jenkinson, M., Smith, S., 2001. A global optimisation method for robust affine registration of brain images. Medical Image Analysis 5, 143–156. doi:10.1016/s1361–8415(01)00036–6.
Jewell, S. W., Hocking, T. D., Fearnhead, P., Witten, D. M., 2019. Fast nonconvex deconvolution of calcium imaging data. Biostatistics 21, 709–726. doi:10.1093/biostatistics/kxy083.
Jo, Y., Faskowitz, J., Esfahlani, F. Z., Sporns, O., Betzel, R. F., 2021. Subject identification using edge-centric functional connectivity. NeuroImage 238, 118204. doi:10.1016/j.neuroimage.2021.118204.
Karahanoglu, F. I., Bayram, İ., Ville, D. V. D., 2011. A signal processing approach to generalized 1-d total variation. IEEE Transactions on Signal Processing 59, 5265–5274. doi:10.1109/tsp.2011.2164399.
Karahanoğlu, F. I., Caballero-Gaudes, C., Lazeyras, F., Ville, D. V. D., 2013. Total activation: fMRI deconvolution through spatio-temporal regularization. NeuroImage 73, 121–134. doi:10.1016/j.neuroimage.2013.01.067.
Karahanoglu, F. I., Grouiller, F., Gaudes, C. C., Seeck, M., Vulliemoz, S., Ville, D. V. D., 2013. Spatial mapping of interictal epileptic discharges in fMRI with total activation, in: 2013 IEEE 10th International Symposium on Biomedical Imaging, Ieee. IEEE. pp. 1500–1503. doi:10.1109/isbi.2013.6556819.
Karahanoğlu, F. I., Ville, D. V. D., 2015. Transient brain activity disentangles fMRI resting-state dynamics in terms of spatially and temporally overlapping networks. Nature Communications 6, 7751. doi:10.1038/ncomms8751.
Karahanoğlu, F. I., Ville, D. V. D., 2017. Dynamics of large-scale fMRI networks: Deconstruct brain activity to build better models of brain function. Current Opinion in Biomedical Engineering 3, 28–36. doi:10.1016/j.cobme.2017.09.008.
Keilholz, S., Caballero-Gaudes, C., Bandettini, P., Deco, G., Calhoun, V., 2017. Time-resolved resting-state functional magnetic resonance imaging analysis: Current status, challenges, and new directions. Brain Connectivity 7, 465–481. doi:10.1089/brain.2017.0543.
Khalidov, I., Fadili, J., Lazeyras, F., Ville, D. V. D., Unser, M., 2011. Activelets: Wavelets for sparse representation of hemodynamic responses. Signal Processing 91, 2810–2821. doi:10.1016/j.sigpro.2011.03.008.
Kinany, N., Pirondini, E., Micera, S., Ville, D. V. D., 2020. Dynamic functional connectivity of resting-state spinal cord fMRI reveals fine-grained intrinsic architecture. Neuron 108, 424–435.e4. doi:10.1016/j.neuron.2020.07.024.
Lindquist, M. A., Waugh, C., Wager, T. D., 2007. Modeling state-related fMRI activity using change-point theory. NeuroImage 35, 1125–1141. doi:10.1016/j.neuroimage.2007.01.004.
Liu, X., Chang, C., Duyn, J. H., 2013. Decomposition of spontaneous brain activity into distinct fMRI co-activation patterns. Frontiers in Systems Neuroscience 7, 101. doi:10.3389/fnsys.2013.00101.
Liu, X., Duyn, J. H., 2013. Time-varying functional network information extracted from brief instances of spontaneous brain activity. Proceedings of the National Academy of Sciences 110, 4392–4397. doi:10.1073/pnas.1216856110.
Liu, X., Zhang, N., Chang, C., Duyn, J. H., 2018. Co-activation patterns in resting-state fMRI signals. NeuroImage 180, 485–494. doi:10.1016/j.neuroimage.2018.01.041.
Lopes, R., Lina, J., Fahoum, F., Gotman, J., 2012. Detection of epileptic activity in fMRI without recording the EEG. NeuroImage 60, 1867–1879. doi:10.1016/j.neuroimage.2011.12.083.
Loula, J., Varoquaux, G., Thirion, B., 2018. Decoding fMRI activity in the time domain improves classification performance. NeuroImage 180, 203–210. doi:10.1016/j.neuroimage.2017.08.018.
Lurie, D. J., Kessler, D., Bassett, D. S., Betzel, R. F., Breakspear, M., Kheilholz, S., Kucyi, A., Liégeois, R., Lindquist, M. A., McIntosh, A. R., Poldrack, R. A., Shine, J. M., Thompson, W. H., Bielczyk, N. Z., Douw, L., Kraft, D., Miller, R. L., Muthuraman, M., Pasquini, L., Razi, A., Vidaurre, D., Xie, H., Calhoun, V. D., 2020. Questions and controversies in the study of time-varying functional connectivity in resting fMRI. Network Neuroscience 4, 30–69. doi:10.1162/netn_a_00116.
Madi, M. K., Karameh, F. N., 2017. Hybrid cubature kalman filtering for identifying nonlinear models from sampled recording: Estimation of neuronal dynamics. PLOS ONE 12, e0181513. doi:10.1371/journal.pone.0181513.
Marrelec, G., Benali, H., Ciuciu, P., Poline, J. B., 2002. Bayesian estimation of the hemodynamic response function in functional mri. AIP Conference Proceedings 617, 229–247. URL: https://aip.scitation.org/doi/abs/10.1063/1.1477050, doi:10.1063/1.1477050, arXiv:https://aip.scitation.org/doi/pdf/10.1063/1.1477050.
Meinshausen, N., Bühlmann, P., 2010. Stability selection. Journal of the Royal Statistical Society: Series B (Statistical Methodology) 72, 417–473. doi:10.1111/j.1467–9868.2010.00740.x.
Moeller, S., Yacoub, E., Olman, C. A., Auerbach, E., Strupp, J., Harel, N., Uğurbil, K., 2010. Multiband multislice GE-EPI at 7 tesla, with 16-fold acceleration using partial parallel imaging with application to high spatial and temporal whole-brain fMRI. Magnetic Resonance in Medicine 63, 1144–1153. doi:10.1002/mrm.22361.
Monga, V., Li, Y., Eldar, Y. C., 2021. Algorithm unrolling: Interpretable, efficient deep learning for signal and image processing. IEEE Signal Processing Magazine 38, 18–44. doi:10.1109/MSP.2020.3016905.
Ongie, G., Jalal, A., Metzler, C. A., Baraniuk, R. G., Dimakis, A. G., Willett, R., 2020. Deep learning techniques for inverse problems in imaging. IEEE Journal on Selected Areas in Information Theory 1, 39–56. doi:10.1109/JSAIT.2020.2991563.
van Oort, E. S. B., Mennes, M., Navarro Schröder, T., Kumar, V. J., Zaragoza Jimenez, N. I., Grodd, W., Doeller, C. F., Beckmann, C. F., 2018. Functional parcellation using time courses of instantaneous connectivity. NeuroImage 170, 31–40. doi:10.1016/j.neuroimage.2017.07.027.
Ortelli, F., van de Geer, S., 2019. Synthesis and analysis in total variation regularization. arXiv:1901.06418.
Patriat, R., Molloy, E. K., Birn, R. M., 2015. Using edge voxel information to improve motion regression for rs-fMRI connectivity studies. Brain Connectivity 5, 582–595. doi:10.1089/brain.2014.0321.
Penny, W., Ghahramani, Z., Friston, K., 2005. Bilinear dynamical systems. Philosophical Transactions of the Royal Society B: Biological Sciences 360, 983–993. doi:10.1098/rstb.2005.1642.
Petridou, N., Gaudes, C. C., Dryden, I. L., Francis, S. T., Gowland, P. A., 2012. Periods of rest in fMRI contain individual spontaneous events which are related to slowly fluctuating spontaneous activity. Human Brain Mapping 34, 1319–1329. doi:10.1002/hbm.21513.
Pidnebesna, A., Fajnerová, I., Horáček, J., Hlinka, J., 2019. Estimating sparse neuronal signal from hemodynamic response: the mixture components inference approach. bioRxiv doi:10.1101/2019.12.19.876508.
Polimeni, J. R., Lewis, L. D., 2021. Imaging faster neural dynamics with fast fmri: A need for updated models of the hemodynamic response. Progress in neurobiology 207, 102174. doi:10.1016/j.pneurobio.2021.102174.
Posse, S., Wiese, S., Gembris, D., Mathiak, K., Kessler, C., Grosse-Ruyken, M. L., Elghahwagi, B., Richards, T., Dager, S. R., Kiselev, V. G., 1999. Enhancement of BOLD-contrast sensitivity by single-shot multi-echo functional MR imaging. Magnetic Resonance in Medicine 42, 87–97. doi:10.1002/(sici)1522–2594(199907)42:1<87::aid-mrm13>3.0.co;2-o.
Preti, M. G., Bolton, T. A., Ville, D. V. D., 2017. The dynamic functional connectome: State-of-the-art and perspectives. NeuroImage 160, 41–54. doi:10.1016/j.neuroimage.2016.12.061.
Raguet, H., Fadili, J., Peyré, G., 2013. A generalized forward-backward splitting. SIAM Journal on Imaging Sciences 6, 1199–1226. doi:10.1137/120872802.
Riera, J. J., Watanabe, J., Kazuki, I., Naoki, M., Aubert, E., Ozaki, T., Kawashima, R., 2004. A state-space model of the hemodynamic approach: nonlinear filtering of BOLD signals. NeuroImage 21, 547–567. doi:10.1016/j.neuroimage.2003.09.052.
Rolls, E. T., Cheng, W., Feng, J., 2021. Brain dynamics: Synchronous peaks, functional connectivity, and its temporal variability. Human brain mapping 42, 2790–2801. doi:10.1002/hbm.25404.
Ruiz-Euler, H. C., Marques, J. R. F., Kappen, H. J., 2018. Nonlinear deconvolution by sampling biophysically plausible hemodynamic models. arXiv:1803.08797.
Sadaghiani, S., Uğurbil, K., Uludağ, K., 2009. Neural activity-induced modulation of bold poststimulus undershoot independent of the positive signal. Magnetic resonance imaging 27, 1030–1038. doi:10.1016/j.mri.2009.04.003.
Schwarz, G., 1978. Estimating the dimension of a model. The Annals of Statistics 6, 461–464. doi:10.1214/aos/1176344136.
Setsompop, K., Gagoski, B. A., Polimeni, J. R., Witzel, T., Wedeen, V. J., Wald, L. L., 2011. Blipped-controlled aliasing in parallel imaging for simultaneous multislice echo planar imaging with reduced g-factor penalty. Magnetic Resonance in Medicine 67, 1210–1224. doi:10.1002/mrm.23097.
Shmueli, K., van Gelderen, P., de Zwart, J. A., Horovitz, S. G., Fukunaga, M., Jansma, J. M., Duyn, J. H., 2007. Low-frequency fluctuations in the cardiac rate as a source of variance in the resting-state fMRI BOLD signal. NeuroImage 38, 306–320. doi:10.1016/j.neuroimage.2007.07.037.
Sporns, O., Faskowitz, J., Teixeira, A. S., Cutts, S. A., Betzel, R. F., 2021. Dynamic expression of brain functional systems disclosed by fine-scale analysis of edge time series. Network neuroscience (Cambridge, Mass.) 5, 405–433. doi:10.1162/netn_a_00182.
Sreenivasan, K. R., Havlicek, M., Deshpande, G., 2015. Nonparametric hemodynamic deconvolution of fMRI using homomorphic filtering. IEEE Transactions on Medical Imaging 34, 1155–1163. doi:10.1109/tmi.2014.2379914.
Tagliazucchi, E., Balenzuela, P., Fraiman, D., Chialvo, D. R., 2012. Criticality in large-scale brain fmri dynamics unveiled by a novel point process analysis. Frontiers in physiology 3, 15. doi: 10.3389/fphys.2012.00015.
Tagliazucchi, E., Balenzuela, P., Fraiman, D., Montoya, P., Chialvo, D. R., 2011. Spontaneous bold event triggered averages for estimating functional connectivity at resting state. Neuroscience letters 488, 158–163. doi:10.1016/j.neulet.2010.11.020.
Tagliazucchi, E., Siniatchkin, M., Laufs, H., Chialvo, D. R., 2016. The voxel-wise functional connectome can be efficiently derived from co-activations in a sparse spatio-temporal point-process. Frontiers in neuroscience 10, 381. doi:10.3389/fnins.2016.00381.
Tan, F. M., Caballero-Gaudes, C., Mullinger, K. J., Cho, S. Y., Zhang, Y., Dryden, I. L., Francis, S. T., Gowland, P. A., 2017. Decoding fMRI events in sensorimotor motor network using sparse paradigm free mapping and activation likelihood estimates. Human Brain Mapping 38, 5778–5794. doi:10.1002/hbm.23767.
Tarun, A., Wainstein-Andriano, D., Sterpenich, V., Bayer, L., Perogamvros, L., Solms, M., Axmacher, N., Schwartz, S., Ville, D. V. D., 2020. NREM sleep stages specifically alter dynamical integration of large-scale brain networks. Iscience 24, 101923. doi:10.1101/2020.07.08.193508.
Tarun, A., Wainstein-Andriano, D., Sterpenich, V., Bayer, L., Perogramvos, L., Solms, M., Axmacher, N., Schwartz, S., Van De Ville, D., 2021. NREM sleep stages specifically alter dynamical integration of large-scale brain networks. iScience 24, 101923. doi:10.1016/j.isci.2020.101923.
Tibshirani, R., 1996. Regression shrinkage and selection via the lasso. Journal of the Royal Statistical Society: Series B (Methodological) 58, 267–288. doi:10.1111/j.2517–6161.1996.tb02080.x.
Triantafyllou, C., Hoge, R., Krueger, G., Wiggins, C., Potthast, A., Wiggins, G., Wald, L., 2005. Comparison of physiological noise at 1.5 t, 3 t and 7 t and optimization of fMRI acquisition parameters. NeuroImage 26, 243–250. doi:10.1016/j.neuroimage.2005.01.007.
Uruñuela, E., Jones, S., Crawford, A., Shin, W., Oh, S., Lowe, M., Caballero-Gaudes, C., 2020. Stability-based sparse paradigm free mapping algorithm for deconvolution of functional MRI data. Proceedings of the Annual International Conference of the IEEE Engineering in Medicine and Biology Society, EMBS 2020-July, 1092–1095. doi:10.1109/embc44109.2020.9176137.
Uruñuela, E., Moia, S., Caballero-Gaudes, C., 2021. A low rank and sparse paradigm free mapping algorithm for deconvolution of fMRI data doi:10.1109/isbi48211.2021.9433821.
Uruñuela-Tremiño, E., Moia, S., Gonzalez-Castillo, J., Caballero-Gaudes, C., 2019. Deconvolution of multi-echo functional MRI data with multivariate multi-echo sparse paradigm free mapping. ISMRM 27th Annual Meeting and Exhibition.
Yeşilyurt, B., Uğurbil, K., Uludağ, K., 2008. Dynamics and nonlinearities of the bold response at very short stimulus durations. Magnetic resonance imaging 26, 853–862. doi:10.1016/j.mri.2008.01.008.
Zhang, X., Pan, W. J., Keilholz, S. D., 2020. The relationship between BOLD and neural activity arises from temporally sparse events. NeuroImage 207, 116390. doi:10.1016/j.neuroimage.2019.116390.
Zoeller, D., Sandini, C., Karahanoglu, F. I., Schaer, M., Eliez, S., Van De Ville, D., 2019. Large-scale brain network dynamics provide a measure of psychosis and anxiety in 22q11.2 deletion syndrome. Biological Psychiatry: Cognitive Neuroscience and Neuroimaging 4, 881–892. doi:10.1016/j.bpsc.2019.04.004.
Zöller, D., Sandini, C., Karahanoğlu, F. I., Padula, M. C., Schaer, M., Eliez, S., Ville, D. V. D., 2019. Large-scale brain network dynamics provide a measure of psychosis and anxiety in 22q11.2 deletion syndrome. Biological Psychiatry: Cognitive Neuroscience and Neuroimaging 4, 881–892. doi:10.1101/551796.
Supplementary Material for Hemodynamic 850 Deconvolution Demystified: Sparsity-Driven Regularization at Work
Supplementary Material for Hemodynamic 850 Deconvolution Demystified: Sparsity-Driven Regularization at Work
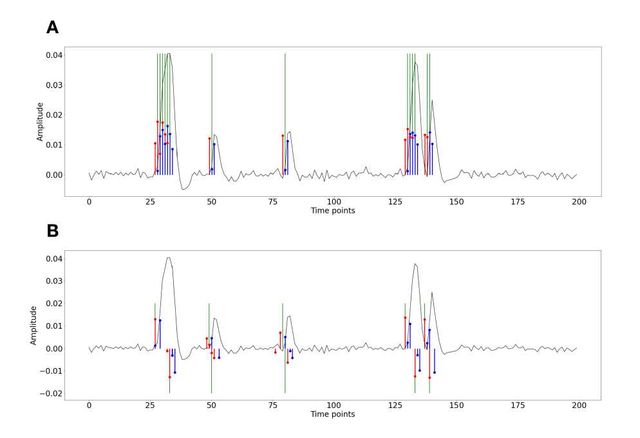
Figure S1: Activity-inducing (A) and innovation (B) signals estimated with PFM (red) and TA (blue) using their built-in HRF as opposed to using the same. The black line depicts the simulated signal, while the green lines indicate the onsets of the simulated neuronal events. X axis shows time in TRs.
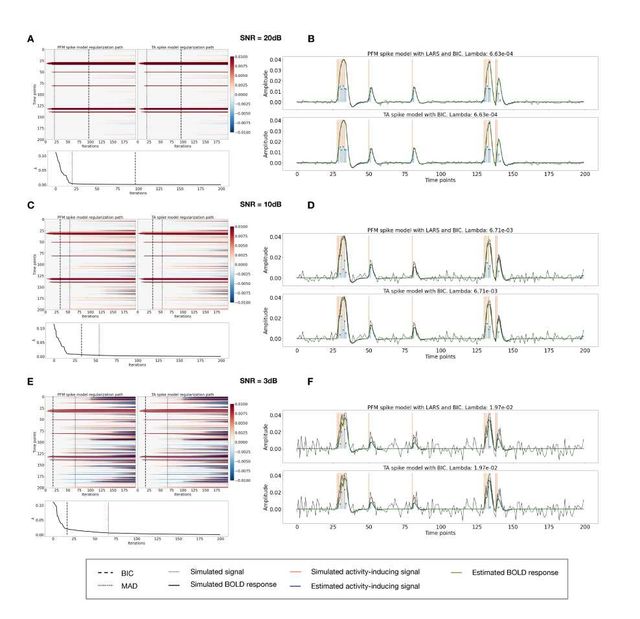
Figure S2: Spike model simulations. (Left) Heatmap of the regularization paths of the activity-inducing signal estimated with PFM and TA as a function of λ (increasing number of iterations in x-axis), whereas each row in the y-axis shows one time-point. Vertical lines denote iterations corresponding to the Akaike and Bayesian Information Criteria (AIC and BIC) optima. (Right) Estimated activity-inducing (blue) and activity-related (green) signals when set based on BIC. All estimates are identical, regardless of SNR.
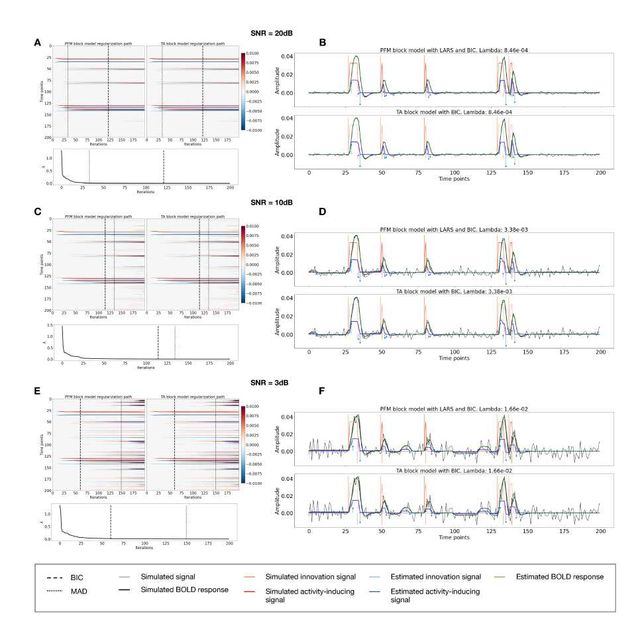
Figure S3: Block model simulations. (Left) Heatmap of the regularization paths of the innovation signal estimated with PFM and TA as a function of λ (increasing number of iterations in x-axis), whereas each row in the y-axis illustrates one time-point. Vertical lines denote iterations corresponding to the Akaike and Bayesian Information Criteria (AIC and BIC) optima. (Right) Estimated innovation (blue) and activity-related (green) signals when is set based on BIC. All the estimates are identical when compared between the PFM and TA cases, regardless of SNR.
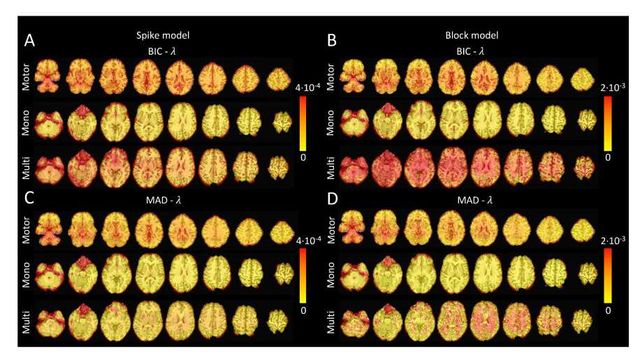
Figure S4: Values of λ across the different voxels in the brain used to estimate (A) the activity-inducing signal (spike model) and (B) the innovation signal (block model) with the BIC selection, as well as (C) the activity-inducing signal (block model) and (D) the innovation signal (block model) with a MAD-based selection. The λ maps are shown for the three experimental fMRI datasets: the motor task (Motor), the monoband resting-state (Mono), and the multiband resting-state (Multi) datasets.
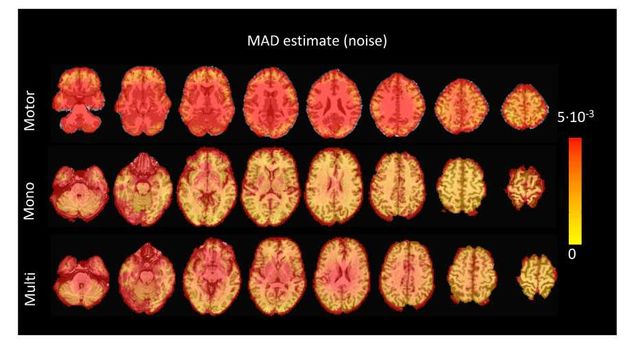
Figure S5: Values of the MAD estimate of standard deviation of the noise across the different voxels in the brain for the three experimental fMRI datasets: the motor task (Motor), the monoband resting-state (Mono), and the multiband resting-state (Multi) datasets.
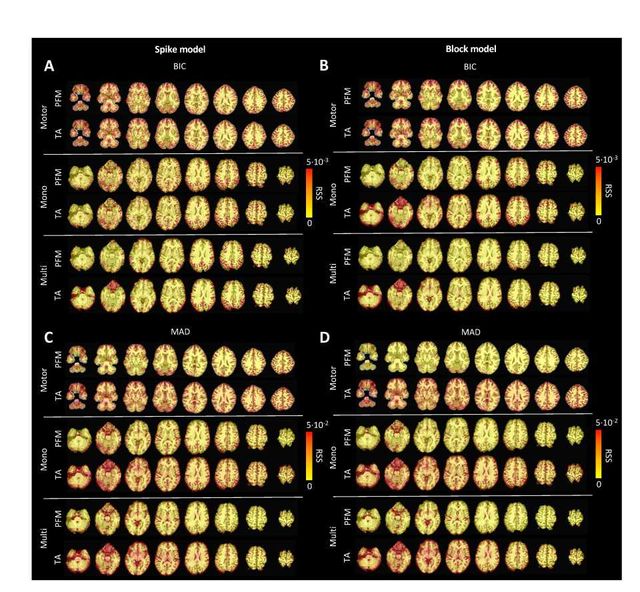
Figure S6: Root sum of squares (RSS) comparison between Paradigm Free Mapping and Total Activation for the three experimental fMRI datasets: the motor task (Motor), the monoband resting-state (Mono), and the multiband resting-state (Multi) datasets. RSS maps are shown for the spike (left) and block (right) models solved with a selection of λ based on the BIC (top) and MAD (bottom) criteria.
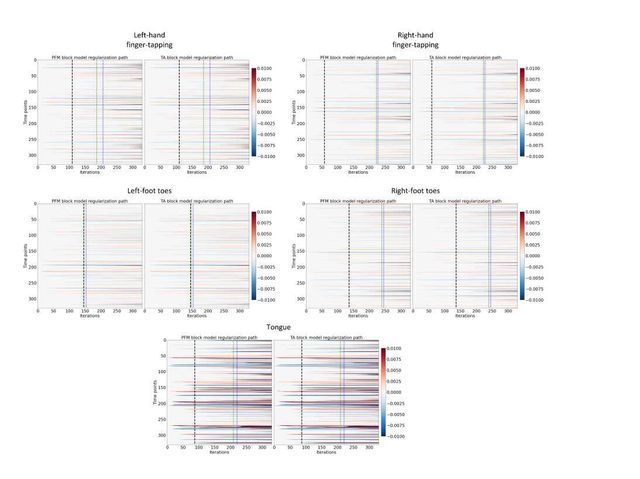
Figure S7: Regularization paths of the innovation signal estimated with PFM and TA as a function of λ (increasing number of iterations in x-axis, whereas each row in the y-axis shows one time-point) for the representative voxels of the motor task shown in Figure 5. Vertical lines denote selections of λ corresponding to the BIC (black), MAD based on LARS residuals (blue) and MAD based on FISTA residuals (green) optima.
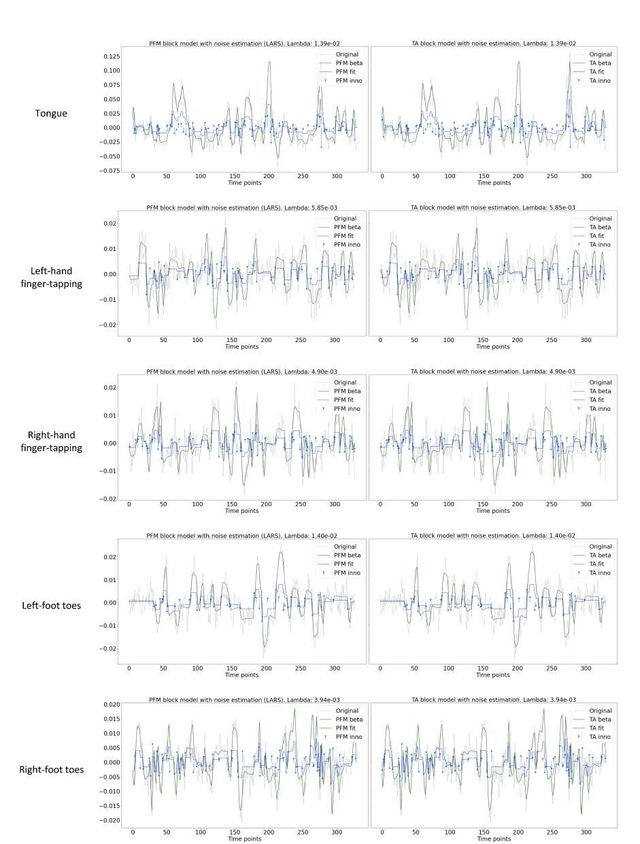
Figure S8: Estimated innovation signal (blue) and activity-related signal (green) for the representative voxels of the motor task shown in Figure 5 with the MAD selection of λ made by TA, i.e., employing the same λ with both PFM and TA.
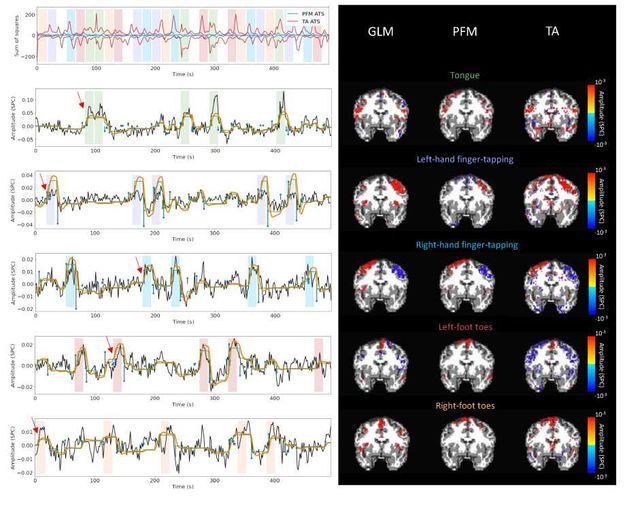
Figure S9: Activity maps of the motor task using a seletion of λ based on the MAD estimate. Row 1: Activation time-series of the innovation signals estimated by PFM (in blue) or TA (in red) calculated as the sum of squares of all voxels at every timepoint. Positive-valued and negative-valued contributions were separated into two distinct time-courses. Color-bands indicate the onset and duration of each condition in the task (green: tongue, purple: left-hand finger-tapping, blue: right-hand finger-tapping, red: left-foot toes, orange: right-foot toes). Rows 2–6: time-series of a representative voxel for each task with the PFM-estimated innovation (blue), PFM-estimated activity-inducing (green), and activity-related (i.e., fitted, orange) signals, with their corresponding GLM, PFM, and TA maps on the right. The maps shown on the right are sampled at the time-point labeled with the red arrows and display the innovation signals at that moment across the whole brain.
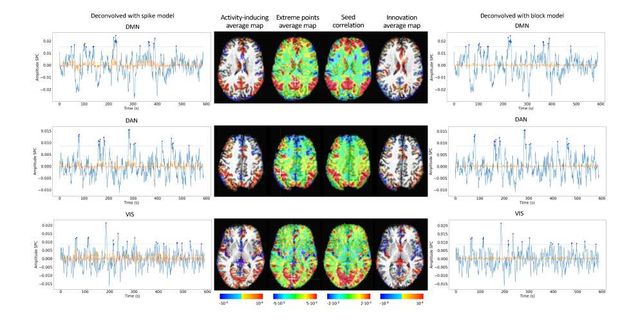
Figure S10: Activity-inducing CAPs (left) and innovation CAPs (right) obtained with the PFM-estimated activity-inducing and innovation signals respectively, using a MAD-based selection of λ. Time-points selected with a 95th percentile threshold are shown over the average time-series (blue) in the seed region (white-cross) and the deconvolved signal (orange). CAPs and seed correlation maps are illustrated in the center.
Metadata
Type of Research Object: